Claude 3.5 Sonnet Multi-Modal Learning . With the release of Claude 3.5 Sonnet, a new generation of AI models, particularly those based on multi-modal learning, has taken center stage in artificial intelligence development. Claude 3.5 Sonnet, developed by Anthropic, represents a groundbreaking innovation in how machines can process and understand information from multiple modalities, such as text, images, and audio, simultaneously. This comprehensive article delves into the architecture, functioning, and use cases of Claude 3.5 Sonnet’s multi-modal learning capabilities.
What is Multi-Modal Learning?
Definition and Importance
Multi-modal learning refers to the ability of AI systems to process and interpret data from multiple input modalities. A modality is essentially a way information is delivered, such as text, images, audio, or video. In traditional machine learning models, one modality is processed at a time, limiting their understanding of real-world scenarios where humans process multiple modalities simultaneously.
Why Multi-Modal Learning Matters
Human cognition thrives on multi-modal inputs: we read, see, listen, and interact simultaneously. To replicate such advanced cognition, multi-modal learning models attempt to integrate and interpret different kinds of information simultaneously. This capability allows AI systems like Claude 3.5 Sonnet to perform tasks that require understanding and combining diverse forms of data.
Overview of Claude 3.5 Sonnet
Evolution of Claude Models
Claude 3.5 Sonnet represents the latest iteration in Anthropic’s Claude series. Previous models were highly efficient at natural language processing (NLP), but with Claude 3.5, the capabilities were expanded to support multi-modal data processing. By integrating different types of data, Claude 3.5 Sonnet enhances its problem-solving ability, making it more adaptable and useful across a wide range of applications.
Key Features of Claude 3.5 Sonnet
- Multi-Modal Capabilities: Able to process and generate insights from multiple data sources, such as text, images, and even audio.
- Contextual Understanding: Enhanced contextual processing to better integrate different data types.
- Faster and More Accurate Processing: Leveraging more powerful cloud infrastructures and distributed computing models.
- Privacy and Security: Advanced safeguards ensuring that user data is processed securely and responsibly.
How Does Claude 3.5 Sonnet Implement Multi-Modal Learning?
Architectural Design
At its core, Claude 3.5 Sonnet employs a transformer-based architecture, but it integrates additional layers that handle multi-modal data processing. Unlike previous Claude versions focused primarily on NLP tasks, this model adds sophisticated neural network layers capable of handling image and audio data.
Multi-Modal Fusion Layer
The multi-modal fusion layer is critical to Claude 3.5 Sonnet’s performance. This layer combines information from different modalities and processes them in a unified manner. For instance, if the model is fed an image and a text description, it can understand how the two relate and use this contextual information to deliver accurate insights.
Attention Mechanisms
Claude 3.5 Sonnet also employs cross-modal attention mechanisms, which allow the model to focus on relevant parts of each modality. For instance, when processing an image and its corresponding text, the model identifies which parts of the image are referenced in the text and vice versa.
Data Preprocessing and Normalization
Before processing, all input data—whether text, images, or audio—undergoes preprocessing to ensure compatibility. Text inputs are tokenized, images are resized and normalized, and audio inputs are transformed into spectrograms for efficient processing. This preprocessing ensures that Claude 3.5 Sonnet can handle multi-modal tasks effectively.
Training and Fine-Tuning
The training of Claude 3.5 Sonnet involves large-scale datasets that include diverse combinations of text, images, and audio. The training process uses a variety of self-supervised learning techniques to help the model understand relationships between different modalities. After initial training, the model undergoes fine-tuning with specific, task-oriented datasets to further enhance its performance for particular applications.
Capabilities of Claude 3.5 Sonnet in Multi-Modal Learning
Text and Image Processing
Claude 3.5 Sonnet excels in tasks that require an understanding of both text and images. For example, it can analyze an image of a product and read a product description to generate accurate summaries or provide detailed analysis based on both data inputs.
- Example Use Case: E-commerce platforms can benefit from Claude 3.5 Sonnet’s ability to process product images and user reviews to generate recommendations or analyze customer sentiments.
Audio-Text Integration
The model can process both audio files and text to provide more nuanced outputs. For instance, it can listen to a speech while reading a transcript and generate a summarized output or a detailed analysis. This capability is particularly useful in sectors like journalism, legal transcription, and content generation.
- Example Use Case: Journalists can feed both a podcast and an accompanying article into the system, and Claude 3.5 Sonnet will generate a detailed summary combining information from both formats.
Video Processing
While Claude 3.5 Sonnet primarily focuses on text, image, and audio modalities, it can also handle video processing to some extent by integrating audio and visual cues. For instance, the model can analyze short video clips, extract key frames, and generate text summaries or transcriptions.
- Example Use Case: Content creators or social media managers can use Claude 3.5 Sonnet to quickly generate captions or descriptions from video content.
Applications of Claude 3.5 Sonnet Multi-Modal Learning
Healthcare and Medical Diagnosis
In the healthcare sector, multi-modal learning is vital for analyzing medical images (such as X-rays or MRI scans) alongside textual medical reports or patient histories. Claude 3.5 Sonnet can assist in diagnosing diseases by synthesizing these different data sources into a cohesive analysis.
- Application Example: A radiologist can input both an MRI scan and a patient’s medical history into the model, which can then generate a comprehensive report, potentially identifying patterns or issues that a single-modality model might miss.
Autonomous Vehicles
Multi-modal learning is key to the development of autonomous driving technologies. By processing visual data from cameras, textual map data, and audio signals, Claude 3.5 Sonnet can aid in making real-time decisions.
- Application Example: An autonomous vehicle system could use Claude 3.5 Sonnet to analyze video feeds from cameras, interpret GPS data, and process audio cues like traffic signals or car horns for a safer and more efficient driving experience.
Education and e-Learning
Claude 3.5 Sonnet can significantly enhance educational platforms by providing multi-modal learning experiences. Students can benefit from receiving content in various formats, including text, images, and audio explanations, leading to a more engaging and holistic learning process.
- Application Example: An e-learning platform can use the model to generate educational content that integrates textual descriptions, audio lectures, and visual diagrams, making the learning process more immersive and effective.
Content Creation and Media
Media platforms can leverage Claude 3.5 Sonnet’s ability to create compelling content by processing data from multiple sources. For instance, journalists can input multimedia sources, such as videos, interviews, and articles, to generate news stories or detailed reports.
- Application Example: A news outlet can use the model to generate articles based on live video reports, transcribed interviews, and raw footage, making the process of content generation faster and more accurate.
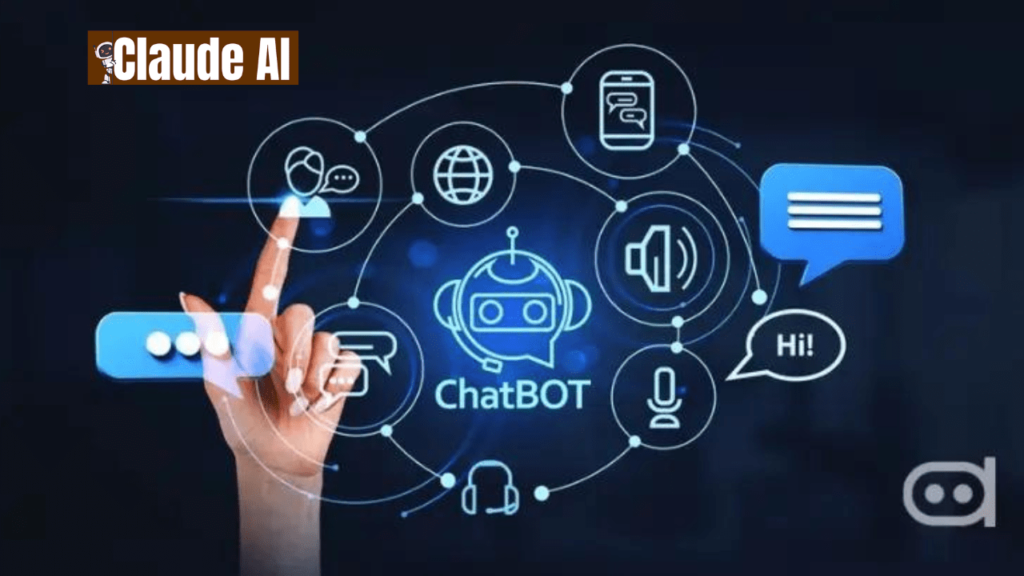
Challenges and Limitations of Multi-Modal Learning in Claude 3.5 Sonnet
Computational Resource Demands
One of the main challenges with multi-modal learning models like Claude 3.5 Sonnet is their high computational requirements. The model’s complexity demands substantial processing power, which can be a barrier for smaller organizations or individual developers.
Data Annotation and Training
Training a multi-modal model requires extensive annotated datasets that encompass different modalities, such as images, text, and audio. These datasets are not only time-consuming to create but also expensive, which poses a challenge for scaling these models for niche applications.
Error Propagation Between Modalities
While multi-modal models are powerful, they are also prone to error propagation. For instance, a misinterpretation in the textual input can negatively affect the processing of the corresponding image or audio. These cascading errors can complicate outputs and require advanced techniques for error correction.
Future of Multi-Modal Learning in AI Models like Claude 3.5 Sonnet
Expansion to More Modalities
The future of multi-modal learning lies in expanding the number of modalities that models like Claude 3.5 Sonnet can process. Beyond text, images, and audio, there is potential to incorporate additional modalities such as haptic data (touch), smell, and other sensory inputs. This would further enhance the model’s ability to interact with the world in a human-like manner.
Improved Efficiency
Ongoing research into more efficient training techniques and model architectures will likely lead to significant improvements in how multi-modal models operate. Techniques like federated learning and quantization could reduce the computational load, making these models more accessible.
Real-World Interactions
The development of AI assistants with multi-modal capabilities could revolutionize how humans interact with machines in real-world scenarios. From personal assistants that understand spoken commands and visual cues to complex decision-making systems that synthesize data from multiple formats, the potential is immense.
Conclusion
Claude 3.5 Sonnet’s multi-modal learning capabilities represent a significant advancement in the field of AI. By enabling the model to process and understand data from multiple modalities—such as text, images, and audio—Claude 3.5 Sonnet offers enhanced problem-solving abilities and is applicable to a wide range of industries. From healthcare and autonomous vehicles to education and media, the future of multi-modal AI models is bright, and Claude 3.5 Sonnet stands at the forefront of this exciting transformation.
FAQs
1. What is multi-modal learning in Claude 3.5 Sonnet?
Multi-modal learning refers to the AI’s ability to process and integrate data from different sources, such as text, images, and audio, to provide a more comprehensive understanding of tasks.
2. How does Claude 3.5 Sonnet handle different data types?
Claude 3.5 Sonnet uses a multi-modal fusion layer and cross-modal attention mechanisms to combine and process information from various data types like text, images, and audio in a unified way.
3. What are the key applications of multi-modal learning in Claude 3.5 Sonnet?
Key applications include healthcare diagnostics, autonomous driving, content creation, e-learning, and media, where the model analyzes data from different sources to provide actionable insights.
4. How is Claude 3.5 Sonnet trained for multi-modal tasks?
The model is trained on large datasets containing various combinations of text, images, and audio using self-supervised learning techniques. After initial training, it is fine-tuned for specific applications.
5. What are the challenges of multi-modal learning in Claude 3.5 Sonnet?
Challenges include high computational resource demands, difficulties in acquiring annotated datasets, and error propagation between modalities that can affect accuracy.
6. How does Claude 3.5 Sonnet ensure data privacy in multi-modal tasks?
Anthropic has integrated advanced privacy safeguards and secure processing mechanisms in Claude 3.5 Sonnet to ensure that sensitive data from multiple modalities is handled responsibly.
7. What is the future potential of multi-modal learning in AI?
The future holds the possibility of integrating even more modalities, such as touch or smell, and improving the model’s efficiency, making multi-modal learning more accessible across industries.